New Algorithm Identifies Disease-Linked Variants in Non-Coding Human Genome Regions
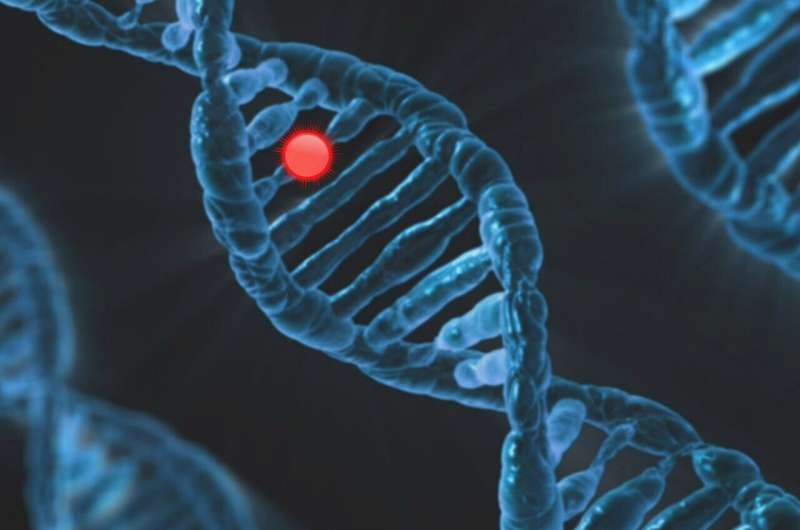
Researchers from the Children's Hospital of Philadelphia (CHOP) and the Perelman School of Medicine at the University of Pennsylvania have developed an innovative algorithm to detect genetic variants in non-coding regions of the human genome that may contribute to disease risk. This breakthrough approach focuses on the vast portions of DNA that do not code for proteins but play crucial roles in regulating gene expression.
While the human genome consists of over 98% non-coding sequences, identifying disease-associated variants within these areas has been historically challenging. Traditional genome-wide association studies (GWAS) have pinpointed broad regions linked to conditions, but isolating the exact variants responsible often remains difficult. Many of these variants are located near transcription factor binding motifs—specific DNA regions where proteins involved in gene regulation, called transcription factors, attach to control gene activity.
The research team employed ATAC-seq, a technique that maps accessible, "open" regions of the genome, and combined it with a deep learning method called PRINT, capable of identifying footprints left by DNA-bound proteins. Analyzing data from 170 human liver samples, they identified 809 specific locations, known as footprint quantitative trait loci (footprint QTLs), which associate genetic variants with the strength of transcription factor binding.
This method allows scientists to see how different genetic variants affect the binding of transcription factors at certain sites, providing insights into how genetic variations influence gene regulation and potentially lead to disease. Dr. Struan F.A. Grant explained that this approach is akin to distinguishing the real culprit in a lineup of suspects, by pinpointing the precise DNA footprint of the disease-causing variant.
The researchers aim to extend this technique to other tissues and organ samples to identify variants that drive various common diseases. According to first author Max Dudek, this approach offers a new way to uncover causative noncoding variants, which could eventually lead to novel treatments.
Published in the American Journal of Human Genetics, this research marks a significant step towards understanding the complex regulatory code of the human genome and its impact on health and disease.
Source: https://medicalxpress.com/news/2025-04-algorithm-potential-disease-variants-coding.html
Stay Updated with Mia's Feed
Get the latest health & wellness insights delivered straight to your inbox.
Related Articles
The Importance of Ethical Oversight in Scientific Research and the Risks of Funding Cuts
Ethical oversight is crucial for scientific progress and public safety. Funding cuts threaten biomedical research, risking delayed treatments and compromised standards. This article highlights the importance of sustained federal support for responsible research.
Advanced Bat Organoid Platform Enhances Pandemic Preparedness Through Novel Virus Research
A newly developed comprehensive bat organoid platform enables detailed study of zoonotic viruses, advancing pandemic preparedness and virus research through scalable, multi-species tissue models.
Genetic Subtypes of T-Follicular Helper Lymphoma Influence Patient Outcomes
Recent research uncovers distinct genetic subtypes of T-follicular helper lymphoma that are associated with different patient outcomes, paving the way for targeted treatments and improved prognosis.
Infertile Women Face Higher Risk of Heart Disease Following Assisted Reproduction
Women with a history of infertility, especially young women and those who undergo fertility treatments, face a greater risk of developing cardiovascular disease later in life. Ongoing research emphasizes early detection and preventive care.