Can AI Predict Your Adherence to Workout Routines? New Research Indicates It Might
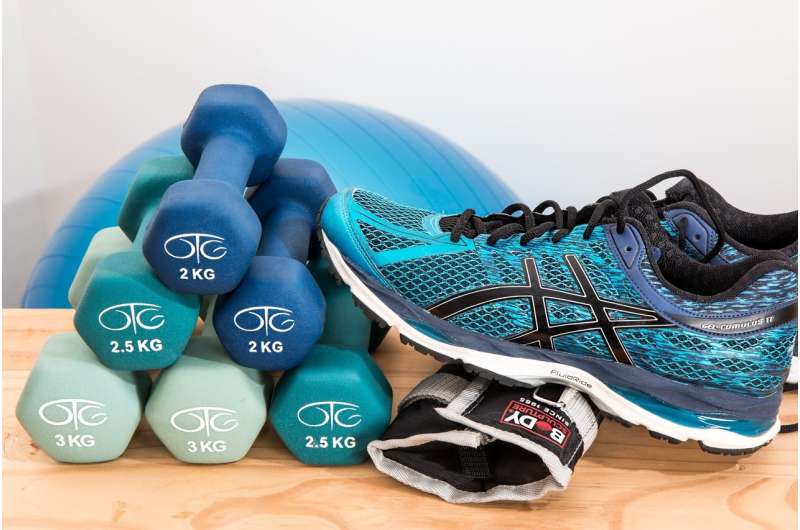
Sticking to a consistent exercise routine remains a challenge for many individuals. In an innovative study conducted by researchers from the University of Mississippi, machine learning techniques are being used to explore what factors influence a person's ability to maintain regular physical activity. The team—comprising doctoral students Seungbak Lee and Ju-Pil Choe, along with Professor Minsoo Kang—aims to predict whether individuals meet recommended physical activity guidelines based on various personal and lifestyle factors.
Analyzing data from approximately 30,000 surveys, the researchers employed machine learning algorithms to identify patterns within the large dataset. These advanced data analytic methods allow for a flexible and detailed understanding of the variables influencing exercise habits, surpassing the limitations of traditional statistical techniques.
Their findings, published in Scientific Reports, underscore the importance of behavioral and demographic factors in exercise adherence. The study highlights that key variables such as sitting time, gender, and education level consistently emerged as significant predictors across different models. Interestingly, educational status was found to be more influential than initially expected, indicating external factors can play a substantial role in health behaviors.
The study utilized publicly available data from the National Health and Nutrition Examination Survey (2009–2018), focusing on adults' self-reported physical activity levels. The researchers considered a broad range of variables, including age, race, income, BMI, lifestyle habits like smoking and alcohol consumption, employment status, sleep patterns, and sedentary time, to assess their impact on adherence.
Despite promising results, the researchers also acknowledge limitations, such as relying on subjective physical activity reports, which tend to overestimate activity levels. Future studies may incorporate more objective measurement tools or explore additional factors like dietary supplement use to enhance prediction accuracy.
Ultimately, these insights could be invaluable for health professionals and fitness trainers in designing personalized workout programs that individuals are more likely to follow long-term. As machine learning continues to evolve, its application in health behavior prediction holds exciting potential for improving public health outcomes.
For further details, see the full study by Choe et al. in Scientific Reports (2025). This research offers promising avenues for leveraging technology to promote sustainable exercise habits.
Stay Updated with Mia's Feed
Get the latest health & wellness insights delivered straight to your inbox.
Related Articles
Innovative Model Overcomes Challenges in Merging Mismatched Geographic Health Data
A new Bayesian modeling approach developed by researchers at KAUST offers a faster, more accurate way to combine mismatched geographic health and environmental datasets, advancing disease mapping and pollution analysis.
The 'Badscopal Effect': High-Dose Radiation Therapy May Promote Growth in Untreated Distant Tumors
New research uncovers the 'badscopal effect,' where high-dose radiation therapy can unexpectedly promote growth in untreated metastatic tumors, highlighting the need for combined targeted treatments.