AI-Powered Digital Pathology Enhances Pediatric Sarcoma Subtyping Accuracy
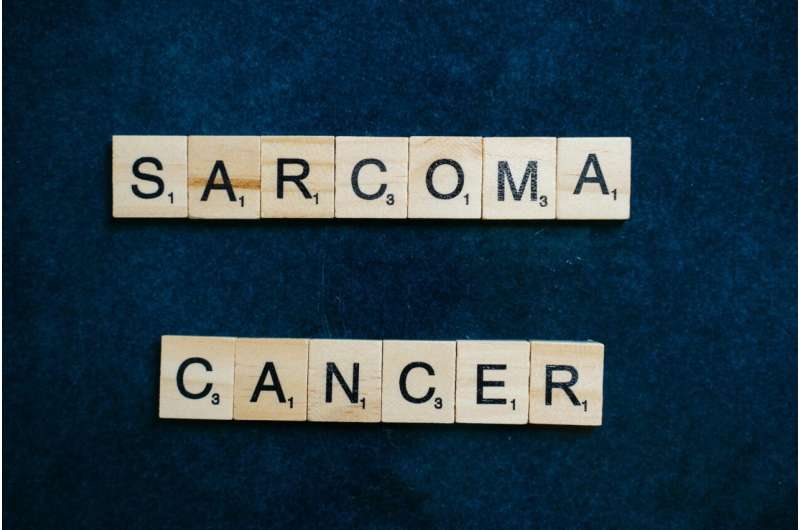
Recent advancements in artificial intelligence (AI) have opened promising avenues in the diagnosis of pediatric sarcomas, a diverse and rare group of cancers originating in various soft tissues such as muscles, tendons, fat, blood vessels, nerves, or surrounding tissues. Accurate classification of these tumors is vital for guiding effective treatment plans, yet traditional methods involve complex molecular and genetic testing, which are resource-intensive and often inaccessible in many healthcare settings.
A groundbreaking study presented at the American Association for Cancer Research (AACR) Annual Meeting from April 25 to 30, showcases how AI models can analyze digital pathology images to classify pediatric sarcoma subtypes with high precision. The study utilized 691 digitized images of pathology slides from renowned institutions, including Massachusetts General Hospital, Yale New Haven Children's Hospital, and St. Jude Children's Research Hospital, covering nine different sarcoma subtypes.
By converting traditional pathology slides into numerical data, AI algorithms identified patterns associated with each sarcoma subtype. The process involved harmonizing images from varied sources to minimize differences due to format, staining, or magnification, then segmenting these images into small tiles for deep learning analysis. The AI models were trained using open-source software and statistical methods to generate feature summaries, enabling precise subtype categorization.
Validation results demonstrated remarkable accuracy: the models correctly distinguished Ewing sarcoma from other types in 92.2% of cases, non-rhabdomyosarcoma from rhabdomyosarcoma in 93.8%, alveolar from embryonal rhabdomyosarcoma in 95.1%, and further classifications with 87.3% accuracy. These findings indicate that AI can reliably diagnose pediatric sarcomas solely from routine pathology images, potentially streamlining diagnostic processes.
This AI-driven approach offers significant benefits, especially for under-resourced healthcare environments. Models are designed to be adaptable, allowing new images to be integrated easily, and can be operated on standard laptops, making advanced diagnostic tools more accessible worldwide.
Despite the promising results, limitations include the relatively modest size of the imaging dataset, reflecting the rarity of pediatric sarcomas. Nonetheless, this collection is arguably the largest multicenter dataset for pediatric sarcoma imaging to date, encompassing various subtypes, locations, and patient demographics. The researchers hope to expand this dataset collaboratively to further improve AI accuracy.
Organized by Dr. Jill Rubinstein of The Jackson Laboratory, this study demonstrates the potential of AI to revolutionize pediatric sarcoma diagnosis, offering faster, more accurate, and widely accessible diagnostic tools, ultimately aiming to enhance treatment outcomes for young patients.
Source: https://medicalxpress.com/news/2025-04-ai-driven-analysis-digital-pathology.html
Stay Updated with Mia's Feed
Get the latest health & wellness insights delivered straight to your inbox.
Related Articles
Newly Identified Ovulation Regulator Shows Potential for Infertility Treatments
A groundbreaking study uncovers the role of Semaphorin 3E in ovulation, offering promising targets for infertility and contraception research.
Innovative Medical Crash Cart Robot Enhances Emergency Team Collaboration and Efficiency
Researchers from Cornell have developed a robotic crash cart to enhance teamwork and efficiency during medical emergencies, reducing stress and improving communication in high-pressure healthcare settings.
Innovative Virtual Reality Training Reduces Infection Risks in Portable Medical Devices
A new virtual reality training program developed by Mass General Brigham enhances infection control practices for portable medical devices, aiming to reduce healthcare-associated infections and improve patient safety.