AI-Driven Brain Scan Analysis Enhances Prediction of Pediatric Brain Cancer Recurrence
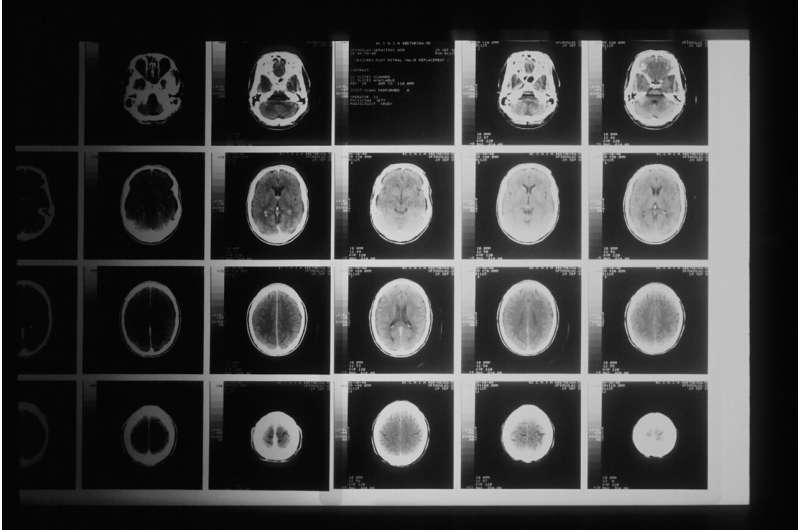
Artificial intelligence (AI) is emerging as a powerful tool in medical imaging, offering new possibilities for early detection and treatment planning in pediatric brain cancer. Researchers from Mass General Brigham, in collaboration with Boston Children's Hospital and Dana-Farber/Boston Children's Cancer and Blood Disorders Center, have developed a deep learning model that analyzes sequential brain scans to predict the likelihood of tumor relapse in children with gliomas.
Gliomas, a common type of pediatric brain tumor, are often treatable with surgery, but the risk of recurrence poses significant challenges. Currently, children undergo frequent magnetic resonance imaging (MRI) scans over many years to monitor for potential relapse, which can be stressful and burdensome for young patients and their families. The new AI approach aims to identify high-risk cases earlier, potentially reducing unnecessary imaging and enabling preemptive therapies.
The study, published in NEJM AI, involved training the neural network with nearly 4,000 MRI scans from 715 pediatric patients. A novel aspect of this research is the use of "temporal learning," where the AI model is trained to interpret multiple scans taken over time, rather than just analyzing a single image. This method allows the detection of subtle changes in tumor characteristics that precede clinical relapse.
The AI model was first trained to recognize the chronological order of scans, learning to detect slight variations over months following surgery. Subsequently, it was fine-tuned to predict whether a tumor would recur based on these changes. Results showed that the model could accurately predict relapse within one year post-treatment, with an accuracy ranging from 75% to 89%. In contrast, predictions based on single scans had only around a 50% accuracy, equivalent to chance.
Providing multiple timepoints improved the model’s accuracy, with four to six scans being sufficient to optimize predictions—a plateau after which more scans did not significantly enhance accuracy. Nonetheless, further validation is necessary before these tools can be integrated into clinical practice. The researchers aim to conduct clinical trials to assess whether AI-informed risk assessments can improve patient management by tailoring follow-up imaging schedules or initiating early intervention for high-risk children.
Lead author Divyanshu Tak emphasized the potential impact of this technology, stating, "This approach harnesses longitudinal imaging data and could be adapted to other conditions requiring serial scans. We are eager to see how it will influence pediatric neuro-oncology and beyond."
This innovative study demonstrates that AI can leverage multiple images over time to improve the prediction of tumor recurrence, marking a significant step toward personalized medicine in pediatric cancer care.
Source: MedicalXpress
Stay Updated with Mia's Feed
Get the latest health & wellness insights delivered straight to your inbox.
Related Articles
Study Finds No Survival Benefit in Reducing Oxygen for Critically Ill Patients
New UK-ROX trial reveals that reducing supplemental oxygen in ICU patients is safe but offers no significant survival advantage, emphasizing the need for personalized oxygen therapy strategies.
Understanding Why Donor Hearts Fail During Cold Storage and How to Prevent It
New research uncovers the molecular causes of donor heart failure during cold storage and offers a promising therapy using existing medication to improve transplant success.
Potential Increase in Obamacare Premiums Expected in Florida Next Year
Expect a major rise in Obamacare premiums in Florida next year as pandemic-era subsidies expire, potentially affecting millions of low- and middle-income enrollees. Learn about the implications for healthcare costs and access.